If you’ve ever asked a car mechanic how long a part will last until it breaks, odds are they shrugged their shoulders. They know how long parts last on average, and they can see when one is close to breaking. But knowing how many miles are left is extremely difficult, even using a supercomputer, because the exact moment a belt snaps or a battery dies is to some extent random.
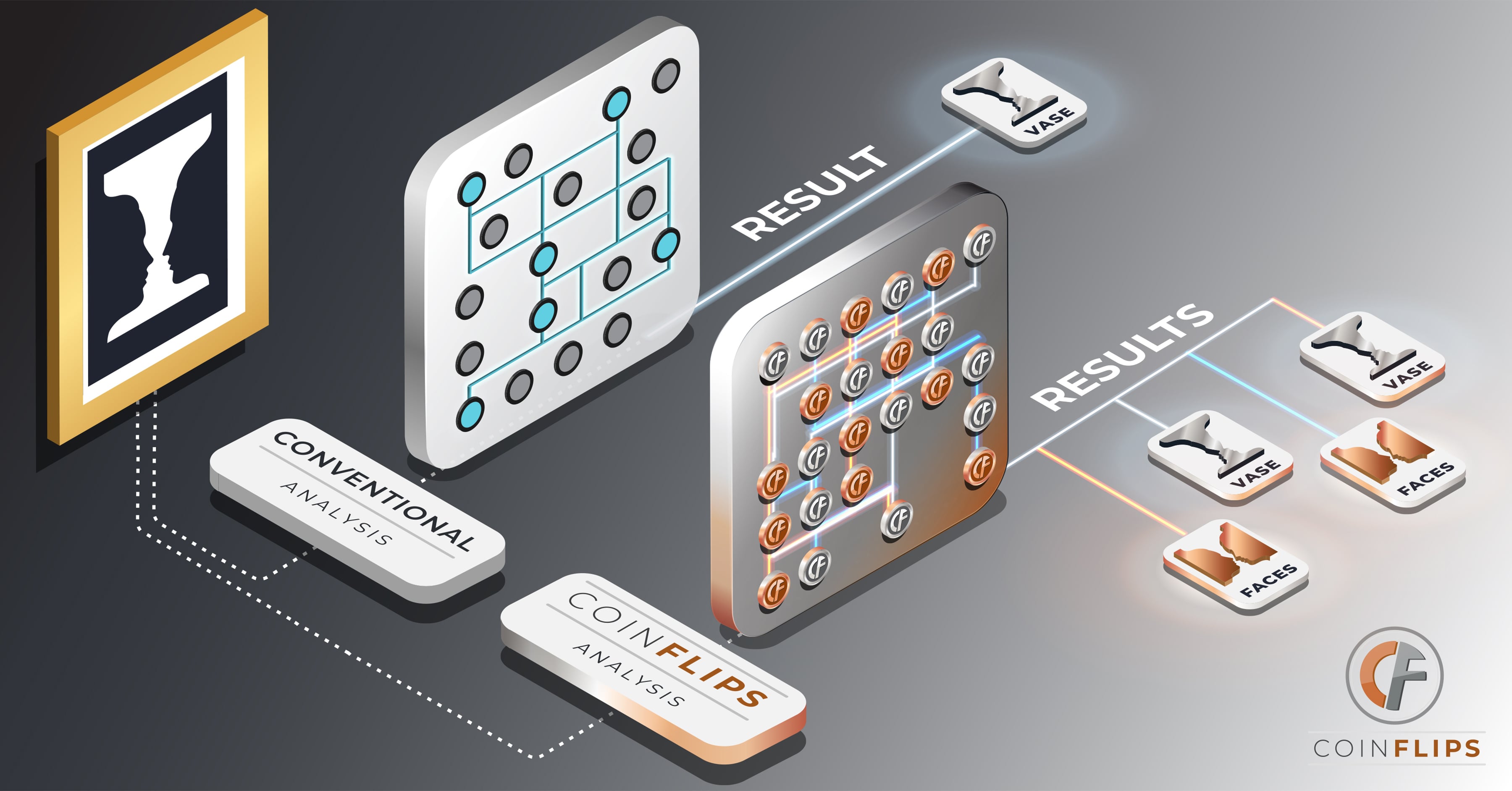
Scientists and engineers at Sandia National Laboratories and several partner universities, including The University of Texas at Austin, are creating a concept for a new kind of computer that can solve complex probability problems like this. They propose that a “probabilistic computer” could not only create smarter maintenance schedules but also help scientists analyze subatomic shrapnel inside particle colliders, simulate nuclear physics experiments and process images faster and more accurately than is possible with conventional computers.
"Probability and uncertainty exist at every level of a computing system, including at the materials, devices, circuits and systems,” said Jean Anne Incorvia, a professor in the Cockrell School’s Department of Electrical and Computer Engineering, who is leading the UT Austin portion of the effort. “We want to take advantage of probability at all these levels."
As part of a new microelectronics codesign research program, the Department of Energy’s Office of Science recently awarded the project $6 million over the next three years to develop the idea. In addition to UT Austin, Sandia will also work with Oak Ridge National Laboratory, New York University and Temple University in Philadelphia.
A codesign microelectronics project involves multidisciplinary collaboration that considers the interdependencies among materials, physics, architectures and software. Researchers also will look at ways to incorporate machine learning methods.
Incorvia and her team are focused on understanding and using stochasticity in magnetic materials and devices for probabilistic computers.
"Here we have an opportunity to make use of new materials and devices as they naturally are, rather than trying to force them to be deterministic,” she said. “A magnet has a magnetization, and, at room temperature, thermal effects can make the magnet randomly switch its magnetization. The magnetism research community, and our team, are interested in making this a feature, not a bug."
The concept for a probabilistic computer runs opposite to how computers are normally built and programmed. Instead of making one that is perfectly predictable, the team wants one with built-in randomness that computes information differently every time.
“To a large degree, and at a great energy cost, we engineer computers to eliminate randomness. What we want to do in this project is to leverage randomness. Instead of fighting it, we want to use it,” said Brad Aimone, a scientist at Sandia who leads the project he and his team call COINFLIPS (short for CO-designed Improved Neural Foundations Leveraging Inherent Physics Stochasticity).
“What if, when I’m communicating with you, I flip a coin?” Aimone said. “If heads, you act on my message; if tails, you ignore it. We want to discover how you can use randomness like this to solve problems where probability is important.”
Concept Modeled After Unpredictable Connections Between Brain Cells
Aimone is an expert in technology that mimics the brain, including machine learning. He got his idea for a probabilistic computer from how brain cells talk to each other:
Inside your brain there are billions of cells called neurons that pass information across trillions of cell-to-cell connections called synapses, Aimone said. Whenever one neuron has a message, it sends a signal to lots of other neurons at the same time. But, only a random fraction on the receiving side carry on the message to more cells. Neuroscientists don’t agree why, but Aimone thinks it could be a reason why brains do some tasks better than computers, such as learning and adapting, or why they use less energy.
To imitate this brain behavior, scientists need to figure out how to generate trillions of random numbers at a time. That much randomness is too complex and takes too much power for computers, said Sandia’s Shashank Misra, who leads the COINFLIPS hardware team.
“We will need to get creative with new approaches, including new materials, atomic-scale control and machine learning-driven designs to generate the sheer volume of randomness needed and to make it useful for computation,” Misra said.
COINFLIPS will also identify tasks that benefit from randomness.
Probabilistic computers are part of a larger effort at Sandia to explore what computers in the future might look like. Researchers around the world have recognized that the rate at which computers are improving is slowing down, Aimone said. To break past the apparent limits of computers, scientists are looking at new, original ways of designing them.